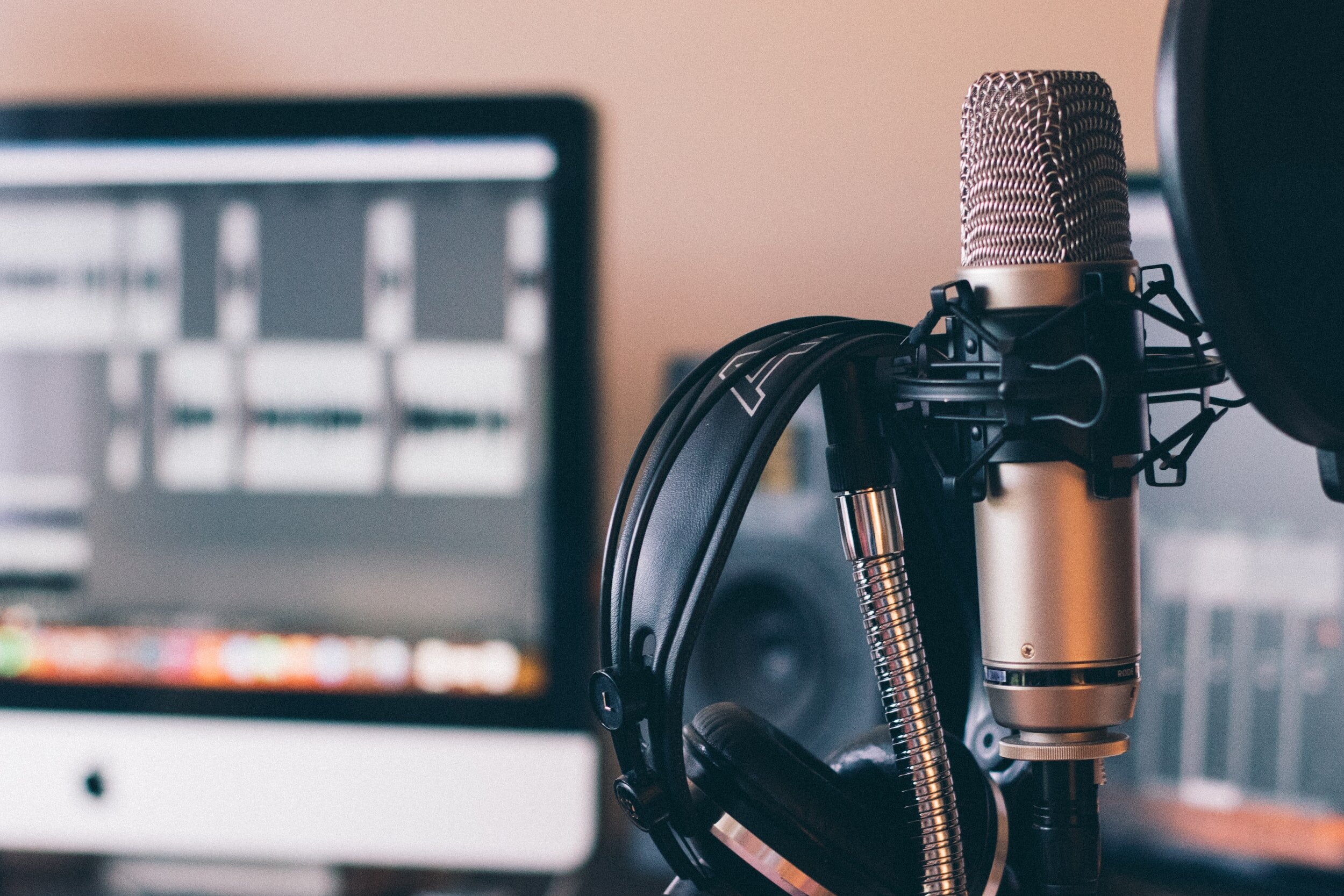
EPISODE 30: LUIS R. SOENKSEN, VENTURE BUILDER IN ARTIFICIAL INTELLIGENCE AND HEALTHCARE AT MIT
Luis R. Soenksen is a serial entrepreneur and medical device expert currently acting as MIT’s first Venture Builder in Artificial Intelligence and Healthcare. His training includes medical device design, development, and manufacturing, as well as clinical testing and deployment of healthcare ventures. Luis has made significant contributions to the fields of bio-design education, bioengineering, tissue engineering, synthetic biology, and artificial intelligence. Luis’ work has been published in several high impact journals such as Science. His research has led to several patented biomedical technologies and collaborations with several start-up companies in Latin America, the US, and Europe. Luis holds a Ph.D. in Mechanical Engineering from MIT, a bachelor’s degree in biomedical engineering from the Monterrey Institute of Technology, and a Master’s of Science degree in bioengineering from Johns Hopkins University.
Episode’s transcript
Julio Martinez: 0:04
Welcome to the Latin MedTech Leaders Podcast. This is a conversation with MedTech leaders who have succeeded in Latin America today. Our guest is Luis Chung . Luis is a civilian entrepreneur and medical device expert, currently acting as MIT's first venture builder in artificial intelligence and healthcare. Luis is an expert in medical device design, development and manufacturing, as well as clinical testing and deployment of healthcare ventures. He's made significant contribution to the field of biodesign education, bioengineering, tissue engineering, synthetic biology, and artificial intelligence. Luis's work has been publishing several high impact journals, such as science and his research has led to several patented biomedical technologies and collaborations with several startup companies in Latin America, the US and Europe. Luis holds a PhD in mechanical engineering from MIT, as well as a bachelor's degree in biomedical engineering from the Monterey Institute of Technology, and also a master's of Science in bioengineering from John Hopkins University. Luis , welcome to the show. It's great to have you here today.
Luis R. Soenksen: 1:13
Thank you , Julio. Thank you so much for receiving me.
Julio Martinez: 1:16
So Luis , could you please briefly tell listeners about your journey to Latin America? How do you get involved with the region?
Luis R. Soenksen: 1:23
Yeah, so I actually, I'm originally from Mexico, born and raised in Mexico City. I did my , um, bachelor's degree at the Monterey Institute of Technology in biomedical engineering, where since the bottom up I started making medical devices primarily in electrical engineering, computer science oriented systems. From there, I work at a couple of different international companies, and I had the fortune to be able to join a cohort of students to do my master's degree at Johns Hopkins University within the Center for Bioengineering Innovation and Design. There I , um, really started my journey in entrepreneurship for medical advisors , uh, building different startups , doing research , uh, about medical research, primarily with great focus on medical devices for both the US and the developing world. I went back to Mexico and work to build infrastructure and build projects within Mexico to serve different international local needs using medical devices including cardiovascular medical devices, intensive care, medical devices and other systems. For example, migraine brain health, diagnostics for breast cancer, you know, a variety of different projects at different stages, which led me to really recognize both the hurdles and the incredible talent that needs to be present all the way in order to, you know, bring up the idea from nothing and lead to regulatory approval and using in real patients to, you know, improve their lives or save their lives. Uh , much more recently, I came back to school and actually did , uh, just finished my PhD at MIT in Boston, well, in Cambridge actually, where I focused , you know, the entirety of my research in a couple of different fields. Uh , primarily synthetic biology, artificial intelligence, and the development of medical devices and teaching engineering. So again, in there the same sort of journey with, you know, even more buildup on the idea of , uh, doing incredible research , uh, you know, that incredible research is actually required nowadays in order to really serve people's lives. Just before, actually, you know, very recently in the last couple of months I've been tasked by MIT as part of this new program called Pro Ventures to launch a variety of , uh, for-profit and non-for-profit organizations, specifically focused on artificial intelligence and healthcare. And so this is an initiative that primarily search for doing accelerated impact based on research developed at MIT and the Boston Cambridge community. And this is primarily what I'm, you know, focusing the a hundred percent of my time now. It's incredibly exciting to be just, you know, deeply involved in this space as this incredible change in the world is happening around us with artificial intelligence.
Julio Martinez: 4:21
Wow, Luis , you certainly have an impressive and fascinating background. <laugh> , I'm proud of having you as a fellow Latin American guest, especially, I'm glad you're involved in artificial intelligence. Seems like , uh, AI will be a big part of the future of healthcare in Latin America. So, Luis , what major epi logical political, social or economic trends do you see in Latin America that are relevant to our discussing about artificial intelligence in healthcare today?
Luis R. Soenksen: 4:52
Yeah, of course. Uh, I mean, how can I put it? So I think there are two sides . Uh, there is the technical trend, right, which, you know, we can talk a lot a little bit more about later, but I think there is a demographic trend that is gonna be even more relevant, the technical aspect of it. So the reality of Latin America and the world is that we are growing at an accelerated pace, and there is no science that population growth is really slowing down. So, you know, in the next 10, 20, 30 years, there is gonna be so many people to serve and, you know, all these people are gonna need healthcare. Yeah.
Julio Martinez: 5:31
And the aging also the aging of the population.
Luis R. Soenksen: 5:34
Exactly. So it's grows demographics, right? So like just in Mexico for example, there is 126 million individuals, right, that they all go around, they work, they get sick, they die, they get born. You know, these 126 million people today, Mexico officials are, you know, challenged with this task of serving a third of the population of the US with a minimum fraction like, you know, at like peanuts compared to what the US spend some healthcare. And so the question here is, how the hell is Latin America actually gonna cope with that challenge of serving, you know, giving quality healthcare to all these people with the limited resources that exist? The only viable opportunity that I honestly see for the next three years is that we start optimizing process in a scalable way to really serve all this population in a way more efficient way. And, you know, reality is that automatization and, you know, like , uh, artificial intelligence is gonna be just a key aspect to make that even possible. Because if we rely, as we have, you know, solely on the non-ag augmented physician, right? And the non augmented clinical systems to serve all these people, there's just not enough time like, you know, and resources and educated resources to achieve it. So I think it's , uh, you know, that component, it's the demographics and the growth and the agent is just like the key aspect. Now, there is , I wanna , uh, actually talk about the challenges though, because the challenges that different countries have are way different. And I think in Latin America, we have a particular set of very interesting challenges that are not, for example, shared by many European countries or the US or Canada, which, you know, go about these unavoidable sort of reality of social injustice, economic disparity, corruption, violence, misinformation that affects, you know, our nations in Latin America, unfortunately. But it's the reality. And so how do you create systems that, you know, can sort of make that leap to give better care at the same time that they're more fair and that they provide, you know, kinda like just improve society as a whole for the region? I believe artificial intelligence, if creative with the right starting points as I'm, you know, kinda like pushing for in the region, I think it's our, you know, prime opportunity to actually fix many of these issues around both demographics and cost, efficacy of healthcare, but also about equality and about giving access to the people that have not been able to have access. You know, the same way cell phones and wireless communications have enabled people to have, you know, to communicate with their families, even in the absence of wired infrastructure, you know, in Africa or in Latin America, the advent of telecommunication technologies, just, it almost like skip a generation, right? Like governments didn't need to build this wired infrastructure anymore because there's no satellites and whatever. Were already serving those, that population , I believe similar things are gonna happen with artificial intelligence in terms of providing, serving more people.
Julio Martinez: 8:40
Very well said. Please . Interesting trends. Thanks. So what have you made so passionate about artificial intelligence and healthcare specifically in the Latin American market?
Luis R. Soenksen: 8:54
Well, first of all, I believe the goal of achieving , uh, general artificial intelligence more, you know, kinda like as a wild dream is probably the single most significant thing. And I know I'm, I'm sounding very anthropocentric here, but I believe it's probably the one of the single, if not the single most important thing that humanity will do. This idea of creating systems that can learn to learn and , and not necessarily because they're gonna oversee those or whatever. It's just that through that exploration, we're really gonna be able to understand how we think and the moment we are able to generate that type of infrastructure or like that type of systems that are just capable of exploring their own problems. And so solving these problems for us and for themselves, I think that's just gonna be absolutely completely transformational for humans, right? And so many people is scared about it , uh, about that other people are skeptical about that type of future. I think regardless, the exploration, the journey towards that goal, I think is just incredibly exciting, right? Like the same type of excitement that I think people in the stone age have when they discover fire and such, like, I think it's gonna take time, but I don't see any fundamental limitation why that shouldn't happen, right? So that's the technical aspect I think, of the excitement to me to understand humans better and to just, you know, even go beyond that , uh, for the benefit of us all . Uh , you know, the universe is so big that I don't believe there is a , you know, absence of space for, you know, many different intelligent agents to, to, to be an exist . Uh, now the second aspect of why I am so excited is that Latin America is just such an incredibly huge untapped space like to, of opportunity in healthcare and ai, that it just blows my mind how little it actually exists today , uh, implementing this space in Latin America. So let me actually explain that. So, you know, with the hundreds of millions of peoples that live today in Mexico, Brazil, you know, Colombia , Venezuela , like Argentina, Chile, everywhere here in Latin America, there is very little that has been done today in order to really utilize the data that has been collected at all. And the other aspect is that I think there is a ton of data that hasn't been even collected at all by governments. And I think that's just like, it blows my mind that companies like Facebook and Google and LinkedIn and Uber, they just can generate these business models that just like basically convince people or full people to give these data and they can then, you know, create value and generate incredible things, right? I think the governments from Latin America and institutions and entrepreneurs alike, I don't think they're fully recognizing kinda like what incredible absence of a stuff exists. So when I see absence, I see opportunity. And so I think in , in Latin America, there is an absence of this infrastructure, and I think companies and governments should feel like, wow, this is a space where we, you know, have a clean slate to do incredible things. And I just believe, you know, that absence of the stuff is an opportunity. And to me, those opportunities are super exciting to be the first to , uh, you know, help create those infrastructures, right? Obviously Mexico and all countries in Latin America, probably they, they have their own local visions about how these should be done and the things that shouldn't be done. And , uh, you know, the , the red tape and, you know, many of these other issues that I mentioned before about corruption or whatever, obviously limit our capacity to even like, think about creating these infrastructures. But this is exactly where innovative entrepreneurs and innovative companies , uh, should direct their thinking efforts, right? Like, how do I convince the ministry of health of Peru to, you know, kinda like create a framework for data sharing that allows the entire population of Peru to be evaluated by researchers around the world in a private, secure, you know, like fair way so that we can create crowdsource models for, you know, the creation of models that help me serve them better, right? And have their taxpayer money be more worth it and be more happy, and then they can even like, pay more taxes because now they are healthier and happier and they work more and whatever. So like these vision, it's an on top opportunity , incredible opportunity for companies to step in, right? To do the investment, right? And to be the first, and when you are first in many markets, and this is gonna be that type of markets, right? First is everything being first is everything, right? That's excitement, both philosophically in terms of the technology and just because this region is like, there's nothing, you see China, right? Uh, you , you know, the ping a doctor and all these systems, I don't know if you have ever heard of this, but like, it's called ping and good doctor, like it's, it , the spelling is really weird, but it's a ping . And then a n good doctor, it's a system that, as I think today, I need to check the numbers, but it's a telemedicine system that is AI enabled , in which right now they already have like 300 million people already subscribed. And it's literally is to create like automatic sort of referrals and automatic prescriptions and automatic like evaluations. This will 300 million plus Chinese already in that like, subscribe to this thing . Like, you know, and I know that , you know , Chinese different laws are different. Like, and I don't personally industry want to do the same ways the Chinese have done, but look at that opportunities, like it could be done and it could be done. It just requires that people step in and stepping in with a little bit of , it's a leap of faith, right? It's an investment that, you know, if done right, it's gonna have a return on investment, right? It's gonna do it, but, you know, people have to step in and work hard and develop that infrastructure so that that return on the investment really happens, right? As a community of , as a regional community. Because that's the only way it's gonna really happen. Now, the third opportunity, I just wanna mention this, and I don't think people necessarily realize is even though Latin America is compounded of, you know, many different countries with many different cultural and ethnic backgrounds, it's actually a pretty, I would say it's a , in general, it's a pretty homogeneous blend of, you know, biologics happening. So like if you look at the genetics of, of, you know, Latin American demographics, like, you know, obviously they all have their difference and some , some may be more related to, you know, Asian heritage or like, you know, even like en endemic , uh, native Americans or Africans coming, you know, kinda like , um, later on in the blending of them and Europeans. But at the same time, I think the mixing that has happened at , at in Latin America overall, it actually permits for countries in the region to actually share their data, to even serve their own countries back. Because, you know, the population in Guatemala, right, as an independent country would , I would say, you know, what happens from a demographic perspective in terms of the predictive models that you can create with data from Guatemala, it's probably gonna be very, you know, useful in the south of Mexico. I mean, right? And so it blows my mind that there is no also, no, and , and this is something that we of course are thinking about, you know, actually launching , uh, at MIT, which, you know, can be at the point of another conversation, but it blows my mind that those frame doors for collaboration internationally within the Latin region and people that go on and have the expertise to develop these models is not in place to really serve the entire , uh, region. And that for me is a huge opportunity. Huge, huge, huge untapped . So super exciting. Yeah.
Julio Martinez: 16:41
Is there anybody who is leading these countries towards these common goals? The Pan-American Health Organization, for example?
Luis R. Soenksen: 16:48
Well, you know, we hope to be the first we will. It is just, anyways, it's gonna happen. Someone's gonna do it. And so we are working actively on, on those types of efforts for sure. And so, you know, I , you know, we, we are, we encourage your audience and abroad to contact us in , you know, and kinda like learn more about what we, we really want to accomplish.
Julio Martinez: 17:10
Excellent. Luis Noble cause I like that. So Luis , what's your opinion on the challenges that Latin America faces in its journey towards in AI enable future?
Luis R. Soenksen: 17:21
Yeah, we touch a little bit about that. So this unavoidable, you know, economic disparities, social injustice, corruption, violence, misinformation, all that is gonna impact the way AI would need to be implemented in Latin America, right? So that it really ensures that it's done for the people by the people. Um , I think more, in a more specific way, I think I , I see four key things that need to happen, the stakeholders level in the region in order for this AI enabled future of healthcare in Latin American to happen. And I believe this is gonna be very specific, and I think they are all challenges, but they are also not only challenges, but actionable statements in the sense that if we solve that challenge, we will be moving towards this future. And so to me, this list, it starts with first building a sizable and high quality digital infrastructure in the region and in all the nations in Latin America . So what do I mean by build a sizable and high quality data infrastructure? Well, so in my mind, evidence-based medicine, which includes, you know, pattern recognition by artificial or naturally intelligent agents, AKA humans can only be accomplished in the presence of large quantities of high quality clinical data, which honestly doesn't exist in the way, you know, I would have hoped that it would exist by now in the region in Mexico, actually, like the biggest, I would say, the biggest forefront. Just to mention, I believe actually Colombia is doing a great job with their dto atos initiative, which is incredible. You actually, you know, you , you just , uh, go browse , uh, dto atos, Colombia , you have this , uh, very nice, it's a little bit hard to explore, but it's a very nice sort of layout of all the data that has been recollected from like different localities in Colombia . It includes like population data, economics, healthcare, you know, social , um, determinants of injustice . Like it has a ton of things that have been measured within Columbia . And, and I think it's a great untapped resource that not a lot of people know about.
Julio Martinez: 19:28
It's worth talking about the Ministry of Health in Columbia , Louis . They have a database called spro, which is perhaps the best database in Latin America to capture patient encounters , procedures, et cetera . The healthcare system in Columbia covers about 95% of the country's 50 million people. So <inaudible> captures in very close to real time all the relevant data related to the country's healthcare system. It's a wonderful thing to have in Latin America, and I hope more countries in the region have move or, or will move towards this data collection efforts.
Luis R. Soenksen: 20:02
Yeah, no, I, I completely agree. I think, I think they're doing the best job in the region. I actually, you know, and this will be controversial, I think it's still insufficient, but I think it's definitely at the forefront there. Now, it's interesting because I think Columbia's at the forefront of execution, but in Mexico, actually, it's very, or at least it's been recognized a little bit in the news as at the forefront in legislation about it. So in fact, Mexico is apparently one of the very first, well, one of the first 10 countries in the world to kinda like publicly government level , publicly commit to sort of like really leveraging artificial intelligence or having a strategy for artificial intelligence in the country, which is very interesting because if you actually see the infrastructure that already exists or that is truly being developed in order to achieve that, it's uh , way behind what , uh, you know, Columbia has, for example. So it's interesting, but I think the desire exists, and again, it's an opportunity and I think it's the moment in which entrepreneurs have to step in and, and just figure it out , uh, how to do it. So the money exists because in fact, for example, just in Mexico, and this is because I'm from Mexico and I know a little bit more about that, but Mexico just committed recently 6.7 billion US dollars to refurbish their social security agency infrastructure to serve . So there is one, there is two public healthcare systems in Mexico, one is called ims , Mexico just embarked in an investment of 6.7 billion, if I remember correctly, US dollars to really build new health infrastructure by 2024. It blows my mind, and this is just , uh, my opinion that none if very little, it's actually intended to be directed into creating a data, a better data infrastructure. This is actually mostly designated for physical infrastructure, which I think public officials in Mexico are not necessarily recognizing that everything is just ever gonna be a little bit more digital and they could potentially serve even more people better at their homes <laugh> than in a new brand new building, right?
Julio Martinez: 22:07
I once heard something like, data is the new currency. Do you agree with that, Luis ?
Luis R. Soenksen: 22:11
I think for sure. I think for governments it shouldn't be perceived as the new currency. The two phrases is , uh, data is the new currency of the world, and data is the new oil of the world. I do believe it's in some capacities, true, I think it's data is becoming ever more valuable as you are, as we're able to do what now researchers call digital phenotyping. So the same way you can have like a genotype and a phenotype in genetics, you can have digital phenotyping now, which is this idea of being able to know a lot of things, non obvious things about people just by looking at the data that we all spread around using, you know, with cell phones that we carry every day , the social media that we look at the sites . So it , it , you know, there is this new, almost new type of phenotype of biological reality, if you will, that is based on that. And it's considered currency because if you know a lot of things about someone, then you can sell them products better. They can convince them to do certain things. It's a salesman's sort of a proposition. Now in governments, in terms of governments in the companies, I think people should think about data more about a new dimensionality of people, right? And basically, you know, you used to have social security numbers, you used to have , uh, bank accounts, how much money where your family, what's your last name? You know, society used to move around the idea of families and the money that you have and whatever. And that's gonna keep being true, but I think you now have to add this extra digital component to people so that you can assess the reputation and their, you know, kinda like their lives at the end. It's , it is just a such a essential part of many people's lives. Now, their digital footprint that I think is just valuable anyways, not necessarily a currency, but, but I agree with you now, let's , uh, that , that's the first point. Build a sizable and high quality infrastructure. The money I think exists, the president of Columbia exists, the presence of the US and other countries exist to build this. So it just needs to happen and the investment needs to happen. And the people in high up with the capacity to make decisions just need to be convinced. Unfortunately, they are not gonna be convinced unless a change from the bottom up happens with, again, entrepreneurs and companies stepping in and saying, this is something that we want to build and we're gonna invest on it. And the moment, you know, a lot of companies and a lot of entrepreneurs start doing that, then governments start to pay attention. And I think that's how it's gonna happen specifically in Latin America where resources are particularly limited from the government perspective. Now, the second point that I wanted to make, it's , uh, the, the second step I think or challenge and then actionable is to develop a scalable nationwide and potentially regional wide governance frameworks for healthcare data. So what do I mean by governance framework? So I don't know if you're familiar of your audience is familiar with the idea of Kaggle , uh, these , uh, Kaggle is this website where you can enter and you can actually look at data sets that people have just put up to do deep learning, machine learning or just data science. So how do you spell Kegel Kaggle , K-A-G-G-L-E , Kaggle , I believe it's a for-profit, but it, it really works pretty much like GitHub. So there is two things. So Kaggle is, it's almost like a competition website where people put data sets that they have anonymized or whatnot, and then people just go there and try to crack the problem that the person or organization that put the data set there. But so it blows my mind, but every single healthcare organization, like big government wise healthcare organization in the us , they already have data sets there to have people solving their problems for free. And these challenges are usually sometimes sponsored, not necessarily in the webpage, but sometimes there is these like events where like people actually compete. And it's actually very interesting because now winning a competition in Kaggle on a interesting data set that is important, it's actually more relevant for the cv, for the curriculum of a computer scientist that publications in peer tier journals. So someone can build a career, like a career having a six figure salary by solving problems with openly available data sets that the government gave. And it's crazy that that framework and very few Latin American organizations, if any, has actually uploaded any anonymized data, therefore sponsoring challenges. So like, I mean, it's just that the community I think doesn't know and it's part of the things that we want to change. And I think this is exactly what I think is great what you're doing here, Julio, interviewing people. Yeah,
Julio Martinez: 26:55
<laugh>, appreciate that. Likewise, <laugh> . Now that we're talking about that quick question here, I'm sorry to interject, but , uh, is there anybody else , uh, any other , uh, institution in the US like MIT doing something similar? Who else is doing something like this?
Luis R. Soenksen: 27:09
We actually have the first, you know, a friend of mine, Marco , incredible Ian researcher , uh, at MIT we want to, to really accomplish in the region by really connecting both top-notch researchers with the communities and the organizations and the people that can make decisions in Latin America. And there's a lot of public officials and people higher up and, you know, people thi can see who's attending that event and everybody's invited to attend. It's still , registration is still open so people can just make it. But regardless of that, I think your question is who else is working on this? And I think we are not the only people working on this at MIT you know, there is many people that from the very beginning, their careers, you know, many years ago have been trying to do things in Latin America. In fact, maybe your audience doesn't know, but the president of MIT since Venezuelan and even other people at MIT, from the secular committees and the , and the people at MIT that that really steer the direction , uh, have been of Latin American heritage at least. And I just mentioned this not because I, not to make a a specific point here, but rather because there's definitely these goal in the back of their minds to help the region obviously has been challenging many levels, but I think, you know, many professors that have been born in, in Latin America that have migrated to the US and MIT have the same feelings and which are just discovering that all these people, you know, they have all these very strong feelings to help and they just need to be catalyzed. And I think this is what really differentiates us a little bit, that we really are trying to create this infrastructure to really catalyze in the right way with the right momentum. Uh, all these people now, other institutions are doing this, so I don't wanna claim we're like the only ones, I believe Stanford, NYU , other universities, top 30 rankings, like they all do have some sort of their perspectives on how to help the region. The issues that I've seen is that most of them have been isolated to usually like research projects with individual institutions instead of like an organized region wise effort. And I think that's exactly why the summit of ai, Latin American is instrumental OMA and the other ones that have really lead that effort. I think that's just the start of doing this the right way. But anyways, the list is one, build a sizable and high quality infrastructure. So we talk about that develop nationwide or regionwide governance frameworks for data healthcare. So how do we figure it out as a region, how to open these data sets in a private way, in a secure way with ethics involved, you know, so that people can, the people in the community can solve these problems for you or, you know, organizations can monetize on their work to solve these issues, et cetera. Right? And third, I would say is for companies and entrepreneurs that want to build stuff like this, my recommendation to you is build your systems solutions to perform highly locally, right? But ambition those systems to scale globally. And what I mean by this, the best problems in the world are shared by many nations, but solutions are almost always local. The best solutions are almost always local, involving local people that know the local problems, that know the culture in there, that know how to really, you know, tackle this in the region, right? But sometimes engineers and companies, sometimes when thinking about the perfect solution for a locality, they lose sight that those same problems may share with the slightly, you know , differences may be shared by many others. And so the opportunity here, right, when achieving systems, when building for systems to perform locally, but scale globally, is that once you have solved the problem that you were originally focused on solving, then you can very easily change something to then serve new markets and potentially monetize on exporting those solutions to the world. So this untapped potential of Latin America to create data sets and create solutions, then it could turn, or for example, imagine that Colombia with all these infrastructure that start creating local solutions, but because the demographics are very similar to other countries around the area, then suddenly Colombia can become almost like an exporter of, of AI solutions that were developed for them, but that now work in the neighboring countries. So you can create this like very thriving marketplace, right? But only if you envision your solutions to scale globally, right? And that's the key. And the fourth, and most , uh, I would say people super forget about this, but please don't, is you have to deploy, measure and repeat. So you deploy your solutions, you measure what happened , and then you reiterate, I believe many people in the medical devices space, specifically in the, in Mexico, they plan like entrepreneurs, they plan for like doing one iteration of their devices just because, you know, the resources are so limited and whatever. But like I have this feeling that it's very risky in Mexico or like Latin America, for example, to do new like innovation medical devices or pharmaceuticals just because they, people plan for just doing one iteration. It's like they have one shot at solving this, and that's just not gonna work. So when people create systems, they have to plan on the resources to do many, many iterations because the version one of their AI model for solving something, it's gonna solve part of it, but it's not gonna be like the Google of , uh, you know, the Google Health of tomorrow. You have to plan for iteration and for measuring. If you don't measure your outcomes, how really it's performing your thing in the wild, then you cannot hope to improve it. And so this is something that the Americans and the Europeans are very good at understanding, but Latin American companies are not very usually good at understanding this. So recommendation finally is like deploy, measure and repeat. Don't think these development of these systems as a one-shot thing is just a start, right? You have to think like, this is the first version one, we're going do version 3, 4, 5, and who knows? Things evolve and then you change markets. You people do do whatever. But deploy, measure, repeat is gonna be fundamental in the space of AI and healthcare , uh, for, for Latin America .
Julio Martinez: 33:15
I really applaud what you and your team is doing in the region. Luis , let's move along here then. So Luis , what's your perception about the regulatory landscape for a AI enabled technologies around the world and more specifically in Latin America? Yeah,
Luis R. Soenksen: 33:30
That's a great question. So , um, you know, I think people that want to enter into this space and want to think about regulatory , uh, processes in general for medical devices, it's , uh, well, actually let me back up a little. AI in healthcare, like primarily from a regulatory perspective, the anticipation is that it's gonna be considered a kind of medical device. So people have think now on AI and healthcare, as you know, if you want to have an intended use that is clearly clinical, right? For using that, I impacts patient . So outside the idea of like, you know, wellness and another product that may not be regulated at all, if you want something that has, let's say FDA approval or covid pre-approval, which is the FDA analogous in Mexico for example, or uh , CE mark in Europe, people have to think, okay, ai, if it has a clinical endpoint for decision support, for, you know, diagnosis for whatever, it's, it's a medical device and therefore it's regulated that way. Now, when thinking about regulation, the most single most important thing that people forget is to very early on fit , kinda like define their intended use. Everything in regulation for medical devices starts with intended use. It's like, what do does this thing has to do? Like what is this thing gonna achieve or what do I want this thing to achieve? And then you fix that, you really think hard about that, like very hard, take your time and then fix that and then say, okay, how are we gonna achieve it? And then there's different regulations that people can read through. And I'm just gonna mention a couple so that people can, you know, can like , uh, hear it in your podcast. But for sure they have to be familiar with this concept of ISO 13 4 85 , which is the main regulation for medical devices in Europe, and then QMS in the us which is a quality , uh, quality management system for medical devices in the us . And then the other piece of regulation that is very relevant is called ISO 14 9 7 1 , which is risk management. And so with those three really you kind of like by sort of knowing very superficially what those really want to accomplish, you can really think about like, you know, in your mind as an entrepreneur, as a company to really sort of start your journey in regulation in terms of strategizing. To really pass this, there is actually a couple of others that I want just to mention. So there's a couple of kind , like regulations, well , uh, more like standards that have been developing them , medical device community that serve to kind like know what things the FDA and others expect from you when you're developing medical devices and medical software. One is the YIEC 66 0 1 dash one from medical electrical equipment. So anything that has a physical electronic thing in medical devices has to follow some of these standards. Then there is also the EC 6 2 3 0 4 , which is software as a medical device. So this actually lays out exactly what in terms of coding and in terms of like , uh, traceability and how you document your code and all that stuff, and how you distribute that code for medical devices that include software. So AI will be obviously included there and the EX 6 2, 360 6 , which inclu , which is human factors, how you deal with the idea of that, you know, all these things actually interact with humans and how humans respond to these systems. So I, I wanted to mention this because I think it's absolutely essential that anyone that is thinking about deploying something in AI and healthcare has to know, has to have like basic knowledge about this. Now the recommendation for me is don't go and read the law all like entirely, just, you know, get yourself familiarized of what that means, right? And then just consult yourself with regulatory experts that just like help you navigate that. But very early on, so like the biggest single issue that actually leads to medical devices not finding regulatory approval is because they don't think about the fact that they have to bypass all these regulations very early on. They basically start doing this thinking once they already did some clinical testing on their device and that it's deemed for failure because part of these quality systems actually in fact require you to document all your process of how you actually led to that solution. Specifically in the risk management sort of law or like , uh, sort of standards. You are encouraged or compelled actually to create a what is called a risk management document in which you document, okay, what are all my modes of failure that this AI system is gonna have? And you actually designed to solve those failure modes, if that makes sense. And if you don't convince that since the beginning, then you are sort of then tasked with having that fully developed system. And then you can like try to fool yourself that you were solving problems that, or risks that. And this is just not, not true. The other thing that I want to mention in term that is gonna be incredibly relevant is two things that are happening in the US and Europe. The first one that happened earlier in Europe is the publishing of this new law called the General Data Protection Regulation , uh, law in 2016, which requires specifically in ai, this new regulation actually requires companies to give users an explanation for any decision that an automated AI system makes. Right? Basically that's deterring designers from relying on what people now call black box ai, right? Many systems in deep learning, you may be familiar about this, they can be highly predictive, but sometimes the connections are so complicated within those neural networks that it's actually very hard to sort of extract out what is the decision tree , if you will, that these systems actually performing order to make a decision, right, on giving care or not care or certain type of care to, to a patient or to another. And the reality here is that all these models, they can fail. They can just be plain wrong because of many different reasons. So let's say that a patient dies because directly because of that information was provided to the physician and that inform physician to do something. So these new regulation really wants to push for making those events more traceable and beyond that to be able to trace back and explain. So what was the rationale of that decision? And so this is actually in my mind, reshaping the industry of medical ai, which is in the need of ways to really create interpretability in this systems, which is right now notoriously difficult to achieve, although a lot of very exciting work is happening in that area. The other aspect in the European regulation is the privacy component to it. So now probably the most stringent, I actually would say nice law from a public perspective on privacy is definitely the European law in which you will see a lot of limitations in terms of clinical data sharing or sharing information that has any identifiable data, including strategies for, you know, differential privacy and other , you know, encryption and other things that need to be achieved. In fact, I've heard from many companies that nowadays you cannot even share data that includes the day or even the month of birth of someone just by combining the demographics or some other data from like social media, whatever. And the year plus the day of birth or the month you can, without having the name, you can kind like just identify, get the social security number or like the zip code of someone. Uh, it's, it's actually not, not that difficult to do. So these privacy laws are now making a little bit more difficult for hospitals and, you know, people just to share that data. But like, but this is just a hurdle that we have to pass and we just have to be , become very creative about how we actually work with that type of regulation to do the, the this the right way. And there there's different strategies such as, again, differential privacy, federated learning, which I'm not gonna explain here, but like people should check out those two terms. The last piece is the stuff that is happening in the US which may be interesting, and I'm talking about Europe and the US just because Latin America is actually gonna follow. It's clear, absolutely crystal clear to me that even though right now there is not really established regulation for AI and machine learning for like medical devices in , in Latin America, you know, historically regulatory bodies in Latin America just follow FDA and Europe on this. And that's, I think it's what's gonna happen. So that's why you have to do know both of them. And so what is happening in the us actually very recently, actually in November of this year, the FDA just released this document, which is a proposed, so almost like a discussion paper, which I think it's , uh, you know, they're asking a little bit for feedback from key opinion leaders on this, but it's called , uh, it's a regulatory framework for artificial intelligence and machine learning, and specifically for modifications of artificial intelligence and machine learning systems in medical devices that have software. So something that you, many of the people in your audience may know is that when you create a medical device before clinically testing it, you have to like freeze it. You have to basically say, I'm not gonna change any single thing from this medical device. 'cause if you test your device and then change, let's say the color of the carcass or one internal cable, then from a regulatory perspective, that device is not the same as the device that was used in the clinical trials. And so then you get into this idea that now you have a system that has a software embedded into it and potentially a machine learning or AI model embedded into it. So traditionally the previous law was thinking, oh, okay, so these models are gonna fix in time, so they're gonna be like fixed and nothing's gonna happen to them. So like that's it. But the reality is that the market is actually moving towards a future in which AI sort of like improves as time goes by as an AI in a cell phone is looking more and more at newer data, it actually can optimize or people is looking at models that optimize themselves to serve better the users. A clear example of these , not for healthcare, but a clear example that you see you're seeing on this is all the text , uh, prediction stuff that you see in Android phones today that actually is improving internally. Uh , many people don't even know about this, but it's actually learning what you do and then you actually, it's able to do something called federated learning and actually improve everybody's cell as phones in every single update. But even within the cell phone , it's like doing these predictions. So you can imagine that that trend is gonna follow in medical devices. So how do you regulate models that are changing over time, which is something the FDA didn't anticipate before. And so these type of regulations is actually giving guidelines and sort of ideas of how this is gonna happen. And , uh, I encourage your audience to check out figure two specifically of this document, which you just, you know, Google ml, ai, FDA and that will be the first thing to do. And apart from that, it really just establishes clear explanation on , on the expectation of quality systems and machine learning practices for AI in healthcare. So I think it's a great resource and Latin America is just gonna follow that. So people that want to really enter into this, they just like, you know, should get used to that and that's just gonna give them a head start .
Julio Martinez: 44:24
Okay. I guess the , the regulatory agencies in Latin America are perhaps, what, three, five years behind the leading ones in other parts of the world?
Luis R. Soenksen: 44:32
Yeah, it's hard to say actually. Like, I would say it depends on the country. Like for example, something that Mexico sort of did is that they created their own sort of like regulatory documentation and guidelines or whatever, but then at some point everything was moving so fast and it happened like a couple of years back, like 6, 5, 7 years ago, they basically started saying, okay, we are, if you have FDA approval, then you can have like automatic approval in Mexico. Like you don't have to even submit like , so the thing that I actually believe part of the reason why they did that is one, to save resources for sure, but like second is just because it's just more cost efficient to let someone like develop these things and take the time and money and effort and whatever to really figure it out. And then somehow, like just by saying, and if you have this approval, then you're automatically approved almost, or whatever. It's almost like updating, like just doing a a like a huge update just by saying like adding a sentence to a law. So I don't believe that's necessarily the right way of doing it just because culture is different. The needs for deploying medical devices are different. And I think these type of behaviors in Latin America actually have led to increased cost of medical care and medical devices and drugs in the region just because people sort of routinely think, okay, if I want to enter to Mexico, I mean I should just get FDA first and then kind of like just go. And that's just a much more inherently costly process just because you have to, in order to achieve that, you have to do clinical trials in the US at least section of them. And so if you had like a , you know, kinda like a framework for making this very clear and easy for people to navigate within the region, I think people, many entrepreneurs would not necessarily require to like, will go to the us . And the other thing is that because this is happening, this is also limiting sort of innovators that may want to be followers for other. So, you know, in medical devices people protect their inventions , uh, the same way as many other fields, but the things that they may not protect their invention in Mexico or in Colombia or in Venezuela or whatever, but that doesn't mean that the needs don't exist in Venezuela or in, you know, Colombia or whatever. So if a company is not patenting in that region, then I would say from an innovation perspective, there's an opportunity for people that want to step in in that country to just either , uh, operate with that freedom to operate <laugh> , not necessarily with a patent, but like with the freedom to operate in that region using kind of like designs that people have disclosed in those patents. Right? And, you know, I encourage obviously to collaborate with the companies that created those inventions just to be, you know, kind of fair and whatever to the people that took the time and the effort and the money to develop this. But you know, if a device is very needed somewhere and it's not serving a specific region, then obviously honestly any , and , and that company that developed that is not economically of financially interested in pursuing those businesses, then it's actually the intent of patents to disclose that information so that other people can follow outside, right, or outside the scope of the , the influence of that patent. Um, so many things to discuss, I guess.
Julio Martinez: 47:30
Yes, please . I feel like this conversation could take a couple days to finish. <laugh>, there's so much to speak about this topic , uh, is is certainly an interesting topic and I'm sure listeners have enjoyed our conversation. Um, so , uh, do you have any final thoughts or muscles of wisdom for AI companies are thinking about venturing into Latin America?
Luis R. Soenksen: 47:53
Yeah , um, I would say take a leap of faith. It's incredible markets to tap in, strategize. However, the way you're gonna accomplish that, and I believe you should do it both bottom up and top down convincing, top down convincing, you know, public health officials having direct conversations with public health officials in the region to sort of align them to your vision, right? Of a good vision for, for your company in the space of AI and healthcare and the entry that you want to have. But also think about bottom up by looking at the communities and the need and this huge social need that your company could serve, right? To the world, companies should not only be responsible for the, the , the wellbeing of the stakeholders themselves, they should be vehicles for social good. And so by having an approach where you take a leap of faith in ai, healthcare and strategize to approach these, you know, create those businesses in Latin America with aligning stakeholders from the top down and the bottom up , you will be poised for success and , uh, you know, initiatives. Here we are at MIT , uh, we'll be very glad to, you know , uh, uh, supporting whatever capacity we want to that . And so, yeah, it's great. Uh, I hope people get excited about this.
Julio Martinez: 49:16
Excellent. Luis , thank you so much for your participation, our show today. I really enjoyed our conversation and I'm sure listeners , uh, did as well.
Luis R. Soenksen: 49:24
Perfect. Super. Thank you.
Julio Martinez: 49:25
Okay, bye-Bye. Take care. Bye.